Data News — must-read articles (mid 2021)
Data News #31 — Must-read articles that have been published over the last year. Learn more about Modern Data Stack, Data Mesh, Data Engineering/Analytics and more.
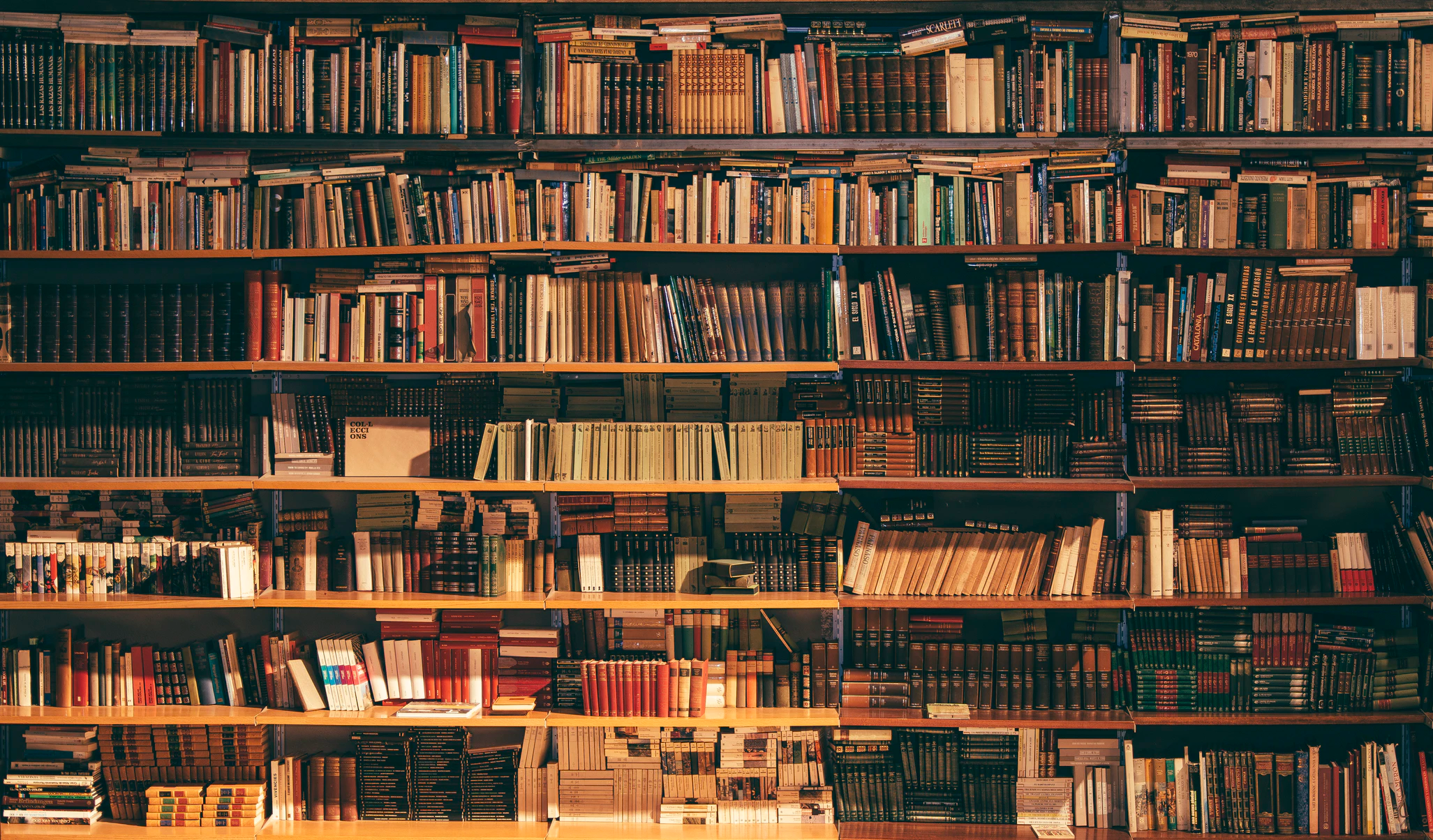
This week I want to share with you a list of articles that have been published during the last year and that are paving the way. The data ecosystem is at cross-roads, a lot of new concepts, new tools and new people are redefining data teams. Below you'll get a first glimpse at what you need to know to understand the trends.
The goal of this edition is to create a photography of last data trends with explanations.
MODERN DATA STACK
The Modern Data Stack: Past, Present, and Future
A must-read article to understand the new way to build data platforms today. How we moved from ETL to ELT using dbt to make the Transformations. Nice writeup by dbt Labs CEO Tristan Handy.
Why the Future of ETL Is Not ELT, But EL(T)
Airbyte CEO, John Lafleur, wrote why the future is mainly about Extract + Load rather than all previous paradigms. If you want to see the opposite side you can read this well-commented discussion on Reddit: "Is it just me or ELT seems over hyped?".
Data & Data Engineering — the past, present, and future
Zack Wilson in echo of the previous articles tries to define where data and data engineering are going with a reminder on the past.
Building The Modern Data Team
Modern Data Stack is trendy, but this is a technical way to see the industry in his thoughts Pedram propose a way to setup the Modern Data Team accordingly.
DATA MESH
What the Heck is a Data Mesh?!
The second big hype in the data ecosystem is around the Data Mesh concepts. As I already showed in a previous news a lake of articles have written on the topic. If you want to fast forward Chris Riccomini can help you.
Building a data mesh to support an ecosystem of data products at Adevinta
This article could help you by sharing an actual journey to implement a data mesh architecture.
PS: As a reminder you can find here the original proposal.
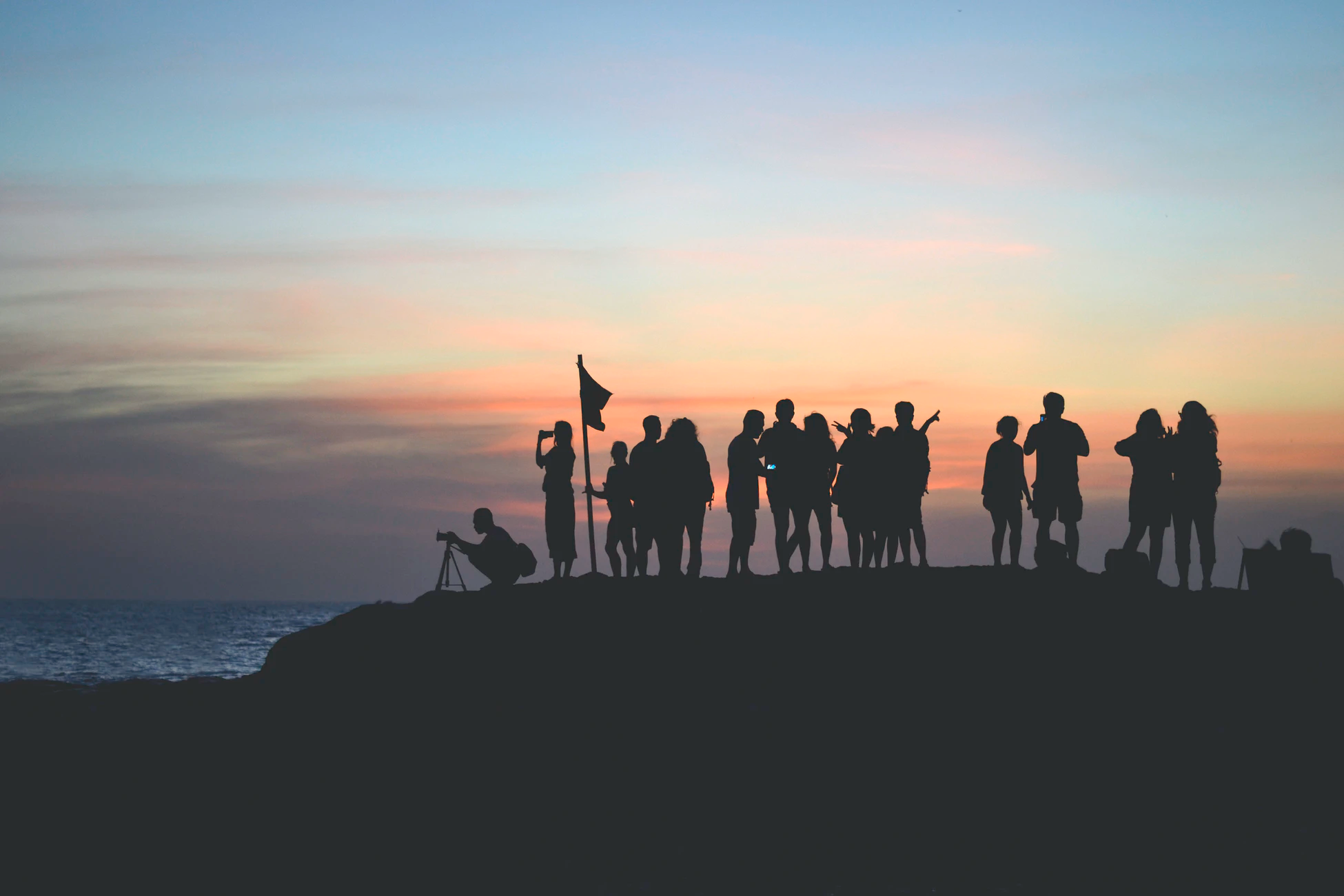
DATA LINEAGE, CATALOGING, OBSERVABILITY, etc.
This year has been a huge milestone in the journey to bring lovable tools to the toolkit of data people. Regarding this I'll refer Future part in Tristan article.
And then I'll wait for the end of the year to see where all the companies are going in term of tooling. But I think that from all the fundraising we got in the last months we are gonna get a lot of features (Airbyte, Alation, Atlan, Bigeye, Castor, Meltano, Monte Carlo, dbt Labs, Soda, etc. — do not hesitate to send me a message to add your company).
DATA ENGINEERING
First I want to share some content formats that are not pure articles but that will help you sharpen your practice and your knowledge of the field:
- Data Engineering Roadmap — DataStack drawn an awesome roadmap to discover all data engineering concepts. A must seen.
- How Data Engineering Works — A YouTube video describing and illustrating in 14 minutes how Data Engineering works.
- Data Engineering Manifesto — I love it. A poster with 9 principles regarding data engineering.
- Data Engineering in 5400-words — Chip Huyen wrote a huge Google Doc with her lecture note on the basics of data engineering.
One Skill Every Data Engineer Needs
I've already featured this article in the newsletter but I found it so true. So here you are. Leo Godin analyses data engineers and what they need the most.
We Don't Need Data Scientists, We Need Data Engineers
Even if the hype over data engineering is just starting we still lack of data engineers. We need these kind reminders. We don't need data scientists, we need data engineers. (Actually, I think we need both).
Introduction to Databases
A README on Github detailing everything you need to know about databases.
DATA ANALYTICS (and TEAMS)
How should our company structure our data team?
This is another way to see data team structure. The article doesn't mention data mesh but ideas are very similar. If you have question around interactions between data internally and externally this is a must-read.
Analytics is at a crossroads
Benn wrote an amazing post here. With everything we saw previously in the post Analytics is at a crossroads. To help you get the larger picture I propose you to read also the manifesto Against SQL and the answer by Pedram For SQL.
What makes a data analyst excellent?
Cassie Kozyrkov, Head of Decision Intelligence at Google, published almost one year ago in Towards Data Science what makes a data analyst excellent (part 2). Starting with misconceptions Cassie shares deep insights.
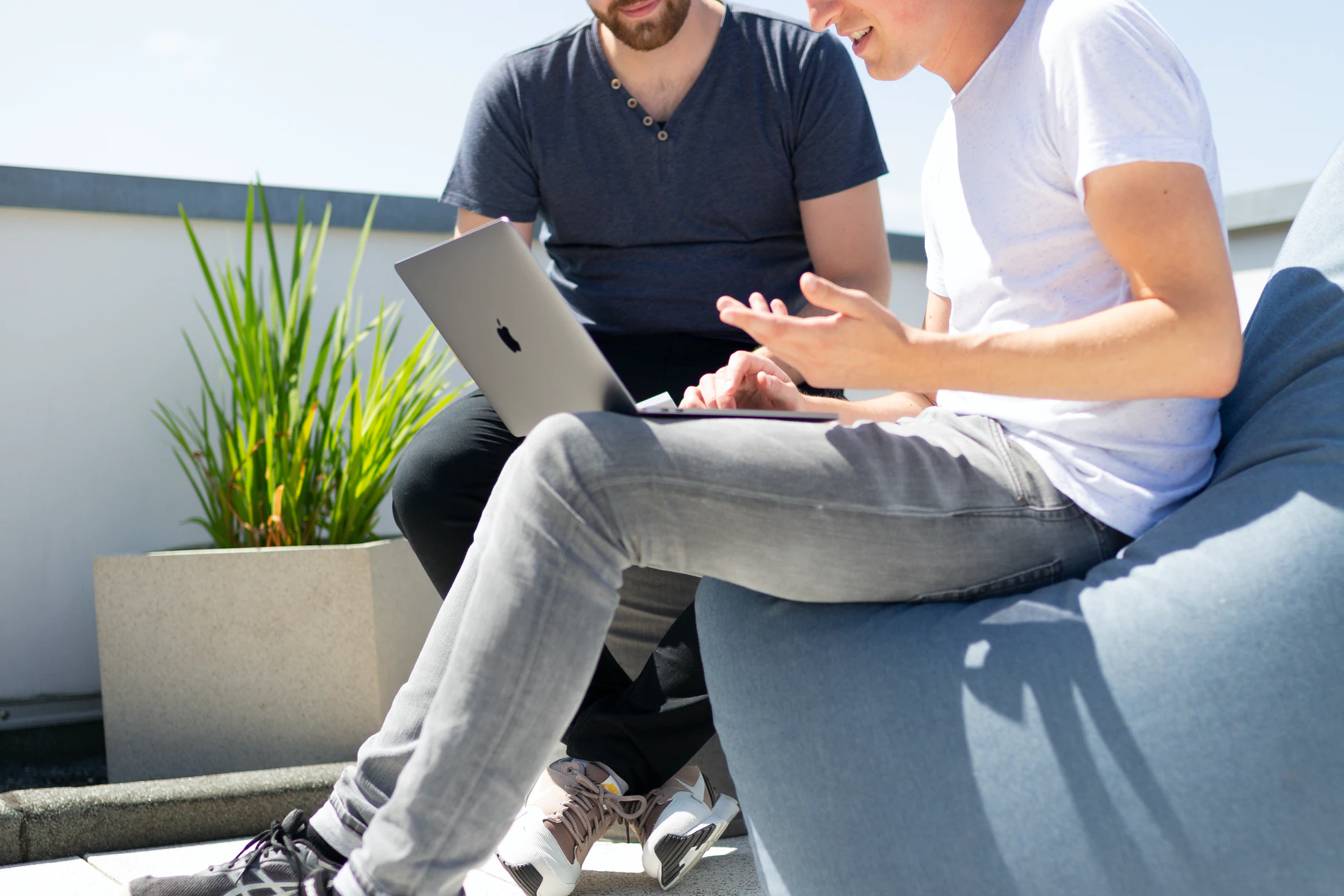
Particular shout-out to Yassine Hamou Tahra and Romain Pierlot for their ideas regarding articles to feature.
See you next week.
blef.fr Newsletter
Join the newsletter to receive the latest updates in your inbox.