Data News — Week 1
Data News #1 — Verb data fundraising, 2022 predictions, data to engineers ratio, using BigTable, side projects, etc.
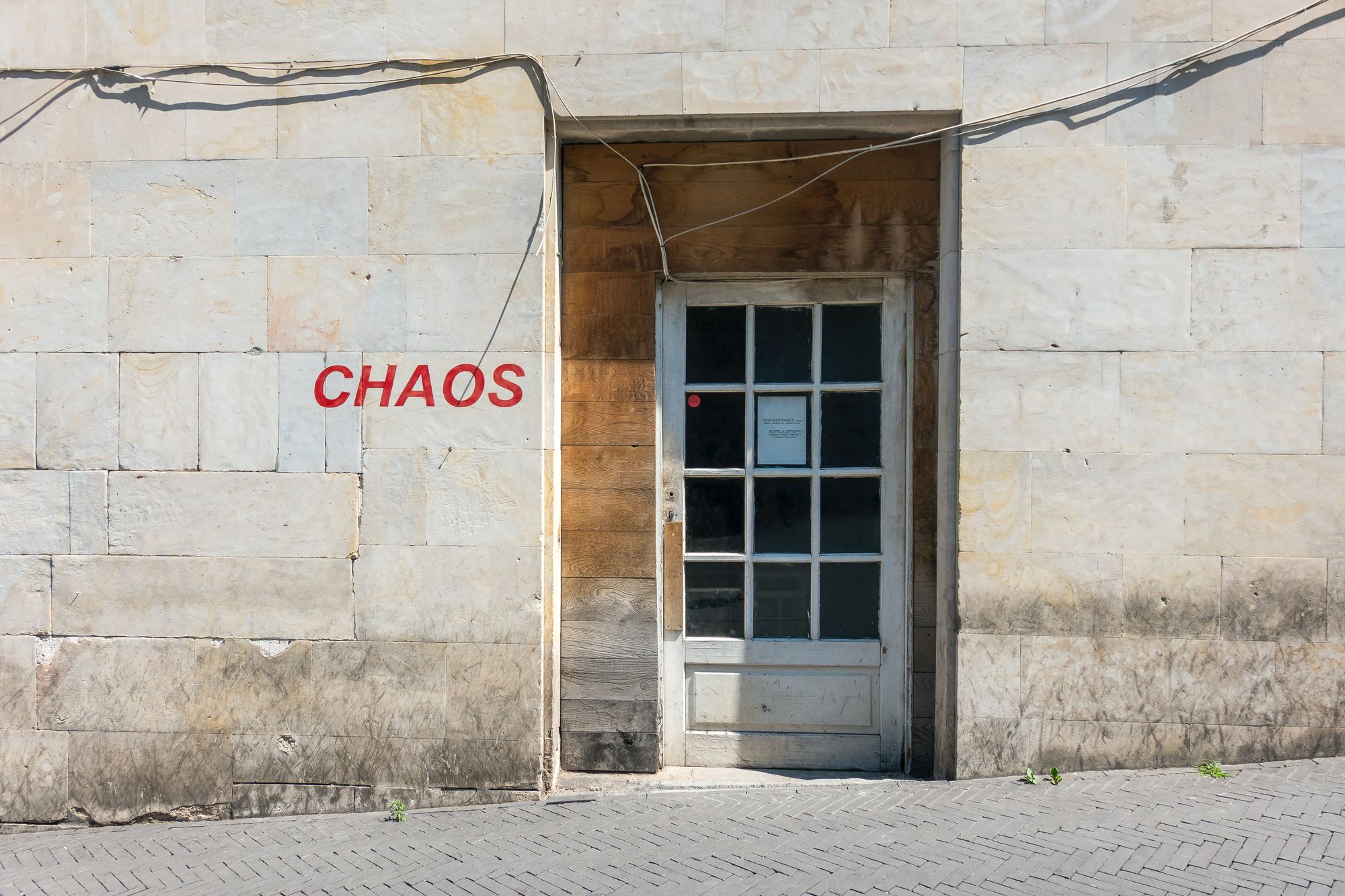
After 2 specials editions the Data News comes back with a longer version than usual, but I hope as good as before.
Data fundraising 💰
After the last crazy year, this new year is starting slowly. I've catch only one fundraising and some fines — but it's a kind of fundraising you know.
- Verb Data raised $3m in funding to continue developing their in-product dashboard tool. Their platform re-create a data platform (with a datalake and a warehouse) from your data in order to give you embed charts via their SDK.
- The French data privacy regulator fined Google €150m and Facebook €60m about dark patterns in their cookie policy. It seems to be the record for France. The CNIL is asking from them to add a "Decline Cookie" button as simple as the "Accept" one. They have 3 months.
2021 summary and 2022 predictions
I did not realize before but a New Year means a lot of best-of articles to finish the year but also some people playing the game of predictions regarding the following 12 months. I'll try summarize what I've seen on both aspect and will maybe play a bit at that game.
First let's talk about databases. Last year has been a huge year regarding databases: huge fundraising and big competition between players. It seems that right now everyone want to store your data. And wow, Snowflake has been elected DBMS of the year — elected means more popular. Bravo, even if I don't understand why we do stuff like this, it probably means something. For the first time in the prize list we have a "Big data" or software-as-a-service player.
So Snowflake, indeed, what a big last year for them. On Ottertune Andy Pavlo did a retrospective on databases. He highlighted the fight between Snowflake and Databricks about speedtest. I highly recommend this post to have a better vision on the space.
This year also saw the talent drain intensifying from Google to others and recently a 17 years of career at Google ML leader joined Snowflake: Tal Shaked.
I've speak a lot about dbt this year, and the community have also written a lot about it. Devoted Health wrote a niece piece about their first anniversary using dbt. They cover how they integrated it with Airflow and the CI/CD concepts going from a POC to more than 1000 models. I've guess that a lot of company took the same path last year.
Salma Bakouk tried to capture the trends that shaped the Modern Data Stack in 2021. Obviously she included tools but also the way we do data, technically but also philosophically. I do agree also with her data mesh and observability were trendy. But to be honest it was only a trendy. We are still waiting for consolidation and real application of it.
What about the Cloud? Erik Bernhardsson wrote in November last year a cool blog about how the cloud will transform and what has changed recently. I really like how he wrote it. The predictions are so true and he excels in the exercise.
YAML will be something old jaded developers bring up after a few drinks. You know it's time to wrap up at the party at that point.
I honestly can wait to be in the future.
Then Microsoft celebrated their Data Science second anniversary. :troll:
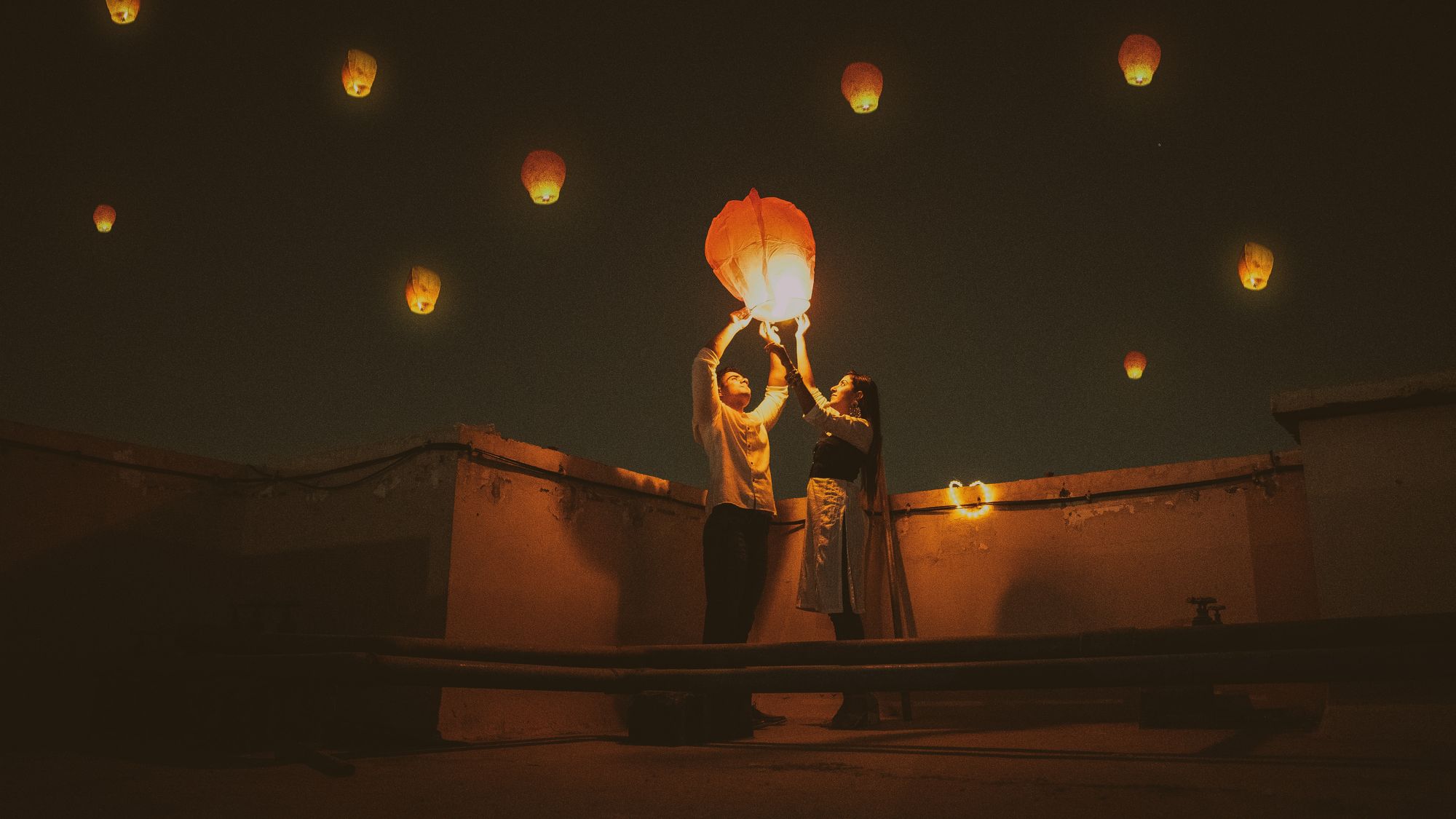
Some wishes I have. I'd like for 2022 less huge fundraising — but I'll continue to track them — and less marketing pressure from data tools and a standardisation of the use-cases in each segment of the MDS. I can mention for instance the observability/quality space that is totally fragmented with a lot, I mean a lot, of different product that are doing almost the same thing with small differences. I share Patrik Liu Tran observations about the data quality space evolutions needed for 2022.
The big issue in this case is for the user. How can we compare those tools when they don't sell for the same use case or don't provide a comparable solution? How can we as user compare them or choose them? Do we need to buy them all?
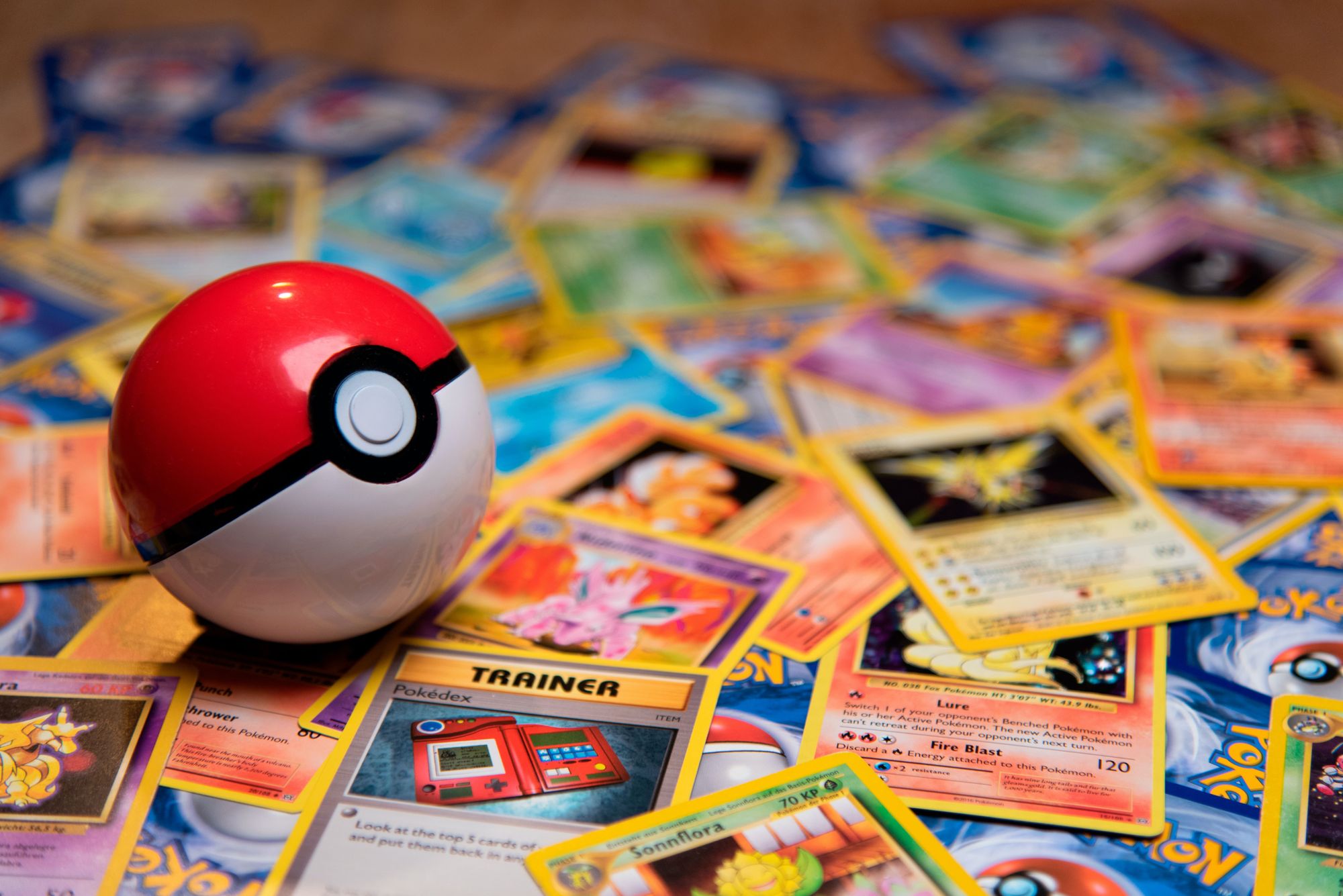
Also now that the transformation segment has been transformed — 🤷♂️ — I hope that in 2022 we will get leaders on the last mile of the data, I mean once the transformation has been done. I'm not yet sure about the metrics layer and how it should evolve or be sold, but still I think that visualisation / exploration layer should be better equipped. And from what I see, a lot of companies are working towards it.
I'd also add two things. First we need better tooling to train juniors or outsiders to data tools, I do teaching about data for the last 6 years and the proliferation of tools make it harder year after year.
Second, this year I'll try to do more data visualisations because I like this. For instance if you want to develop a TV tuner recorder that does speech-to-text to analyse words occurrence in TV morning shows[FR] you can do it. To help you having ideas look at open datasets, we have more open data as the Open Data Maturity index reports in Europe.
Data to engineers ratio: A deep dive into 50 top European tech companies
Mikkel did a huge work looking at the ratio between data and engineering teams by looking at the number of currently open roles in 50 EU tech companies. To be honest this is a good ratio to have a look at but I think this is difficult to get some conclusion from it
Because it does not take into account the current situation and the data roles are so diverse that it will include outliers. Obviously there isn't a lot of companies where data is larger than engineering so it gives a good trend.
But in the end, why should we oppose engineering and data? Isn't the data engineer the final evolution of the software engineer? 🙃 João proposed us a vision going that way following what decentralization offers to us. Software engineers will evolve and own data publication when doing PLT instead of ELT and probably data engineers specificities will disappear.
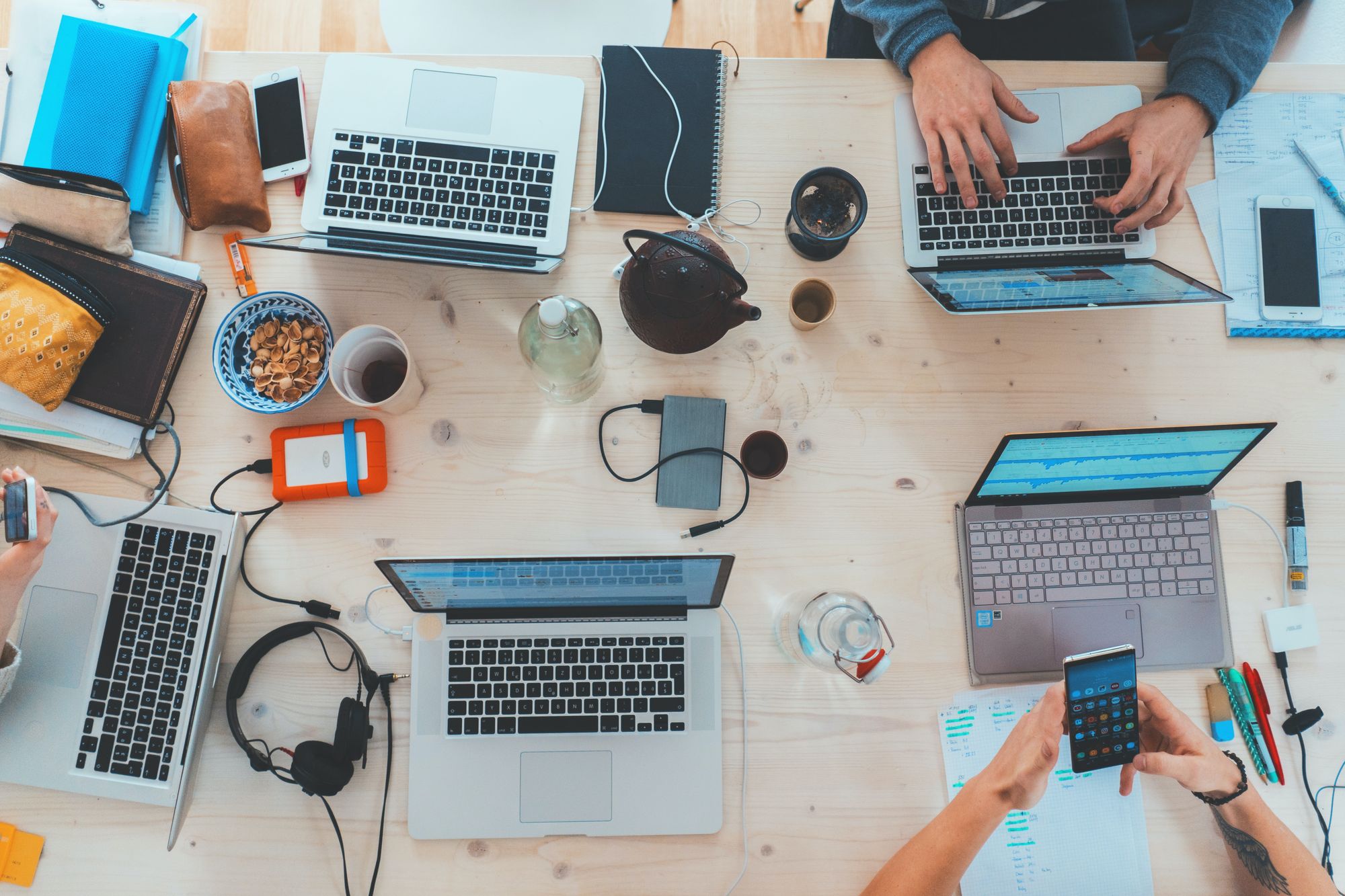
Airbyte journey with Kubernetes
Airbyte team detailed how they used Kubernetes to scale their workloads. The post is quite long and details what major challenges they faced while doing it. Like using socat to redirect stdio between pods.
Were we all using Airflow wrong?
If you remember this popular post about how we were all using Airflow wrong — tbh I still disagree with this post because I still think that the strength of Airflow is not only his scheduler but also his Python modularity. Locale.ai team is saying that now with the KubernetesPodOperator it is fixed. I got a bit clickbaited by the post because actually they just used KubernetesPodOperator.
Choosing the best schema to improve Google BigTable performance
If you think about doing a Feature Store or if you do BigTable this post by Algolia tech may help you designing your BigTable data schema. BT is something really specific for people not use to it — like HBase — and the post is a good starter for you to understand it. They explain very well how they merge the batch and the speed layers thanks to BT.
Fast News ⚡
- Artificial Intelligence in Magic The Gathering — this is a good side project if you love card games. Gabriel developed a Streamlit application to explore MTG cards.
- How to Use TomTom Data for Predictive Modeling — Another idea of side project, TomTom team detailed how you can use their data to predict traffic.
- Sorry Ladies… This Is Still a MAN’s World! — details why AI is still not gender-neutral.
- Analytics with SQL: What keeps them coming back? — Using arrays in SQL to write clickstream analysis.
- MySQL character sets explained – why utf8 is not UTF-8 — I really like database explaination posts. Even if it's MySQL and I stoped using it 10 years ago it still demystify stuff.
- Use your Airflow metadata — That is a good inspiration post for people using Airflow. Don't let the data sleep there. Use it to create observability on top of your Airflow instance.
Graphext team used the data from the newsletter to visualise it in their tool. This is nice to see how the articles I've been featuring for the last months are clustered. Thank you a lot for this contribution!
blef.fr Newsletter
Join the newsletter to receive the latest updates in your inbox.