Data News — Week 22.31
Data News #22.31 — Rill and LiveEO fundraising, have you seen my privacy?, the data meh, best practices and learning and the best fast news of all time.
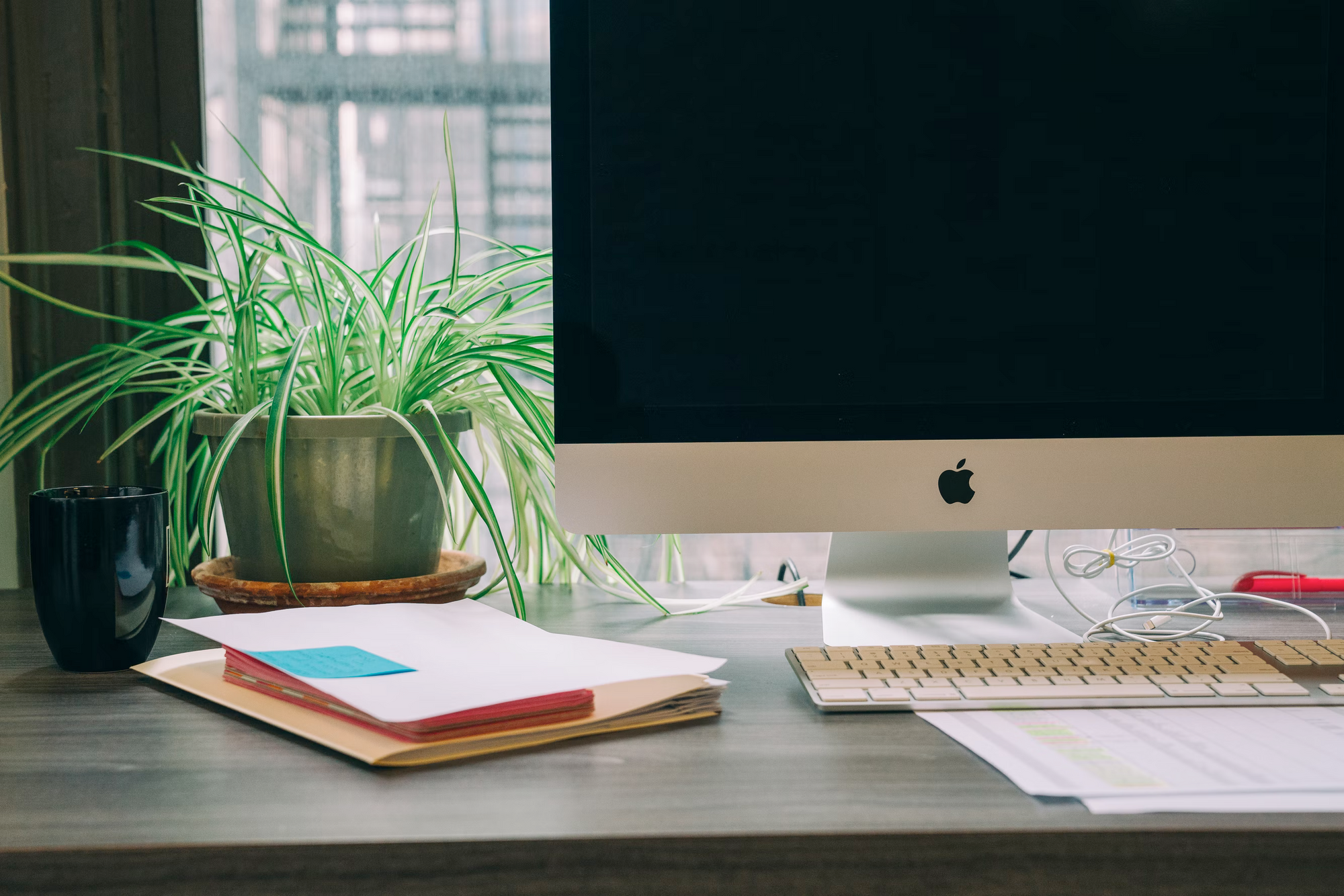
Hey, I was travelling this Friday so I couldn't finish the newsletter on time. But here you are. I hope you will enjoy. August is really the middle of the year for me. Here a quick summary on my plans:
- Almost 2 years since I've started as an independent and I've just turn 30 last week. I'll prepare a post on my data engineering freelance journey. Right now I'm mainly working for the French Ministry of Education.
- Next week I'll move to Berlin (saying a small au revoir to Paris)
- I plan to increase the content I create starting in September: more videos, mentoring and training. If you like my content, you can consider becoming a paying subscriber (it's 45€/year) and it'll allow me to stay independent.
- I want to develop small tools to help data professionals: the dbt-helper extension, dbt-doctor CLI, a data freelance community, a job board here on the blog, etc.
If there is something you would like to see from me, do not hesitate to hit reply 📩.
Data fundraising 💰
- Rill raised $12m in seed round — which is huge for a seed round — to bring a new vision to business dashboards. From the GIF on their landing page it looks promising: a SQL-based BI tool with real-time database behind. Under the hood it uses a combination either DuckDB — for the developer version — and Druid for the enterprise one.
- LiveEO raised $19.5m in Series B. This isn't directly related to a data product but it showcases where we are today in term of AI use-cases. LiveEO monitors the ground thanks to satellite images to help prevent wildfire — and we got a lot this year — or to detect intruders. When these technologies are used for the good it can be awesome but what's the ethical line to not cross?
Have you seen my privacy?
The General Data Protection Regulation — GDPR — has been originally published in 2016. Since then, other regulations followed: Data Protection Act 2018, POPIA, LGPD, PIPEDA, Data Privacy Act, CCPA. I'm not qualified to evaluate these laws, still I feel this is a good start.
But, there is an elephant in the room. Implementing the GDPR is close to the twelve labours. When it comes to the data team there isn't a proper word to describe the size of the elephant. I can't pinpoint a thing to change to implement the GDPR in the modern data stack, everything needs to change. Data leaks everywhere.
Salma tried to summarize all the rights at stakes regarding GDPR. She also mentions these 12 items to understand the GDPR. I also decided to do this edito because this week TotalEnergies has been fined €1m (😂) because they created a form without opt-out. In addition Criteo will, maybe, face €60m sanction also because of consent.
But behind this smoke screen about consent, while companies and organisations are using satellite, cameras, social networks, etc. to detect stuff, have you seen my privacy?
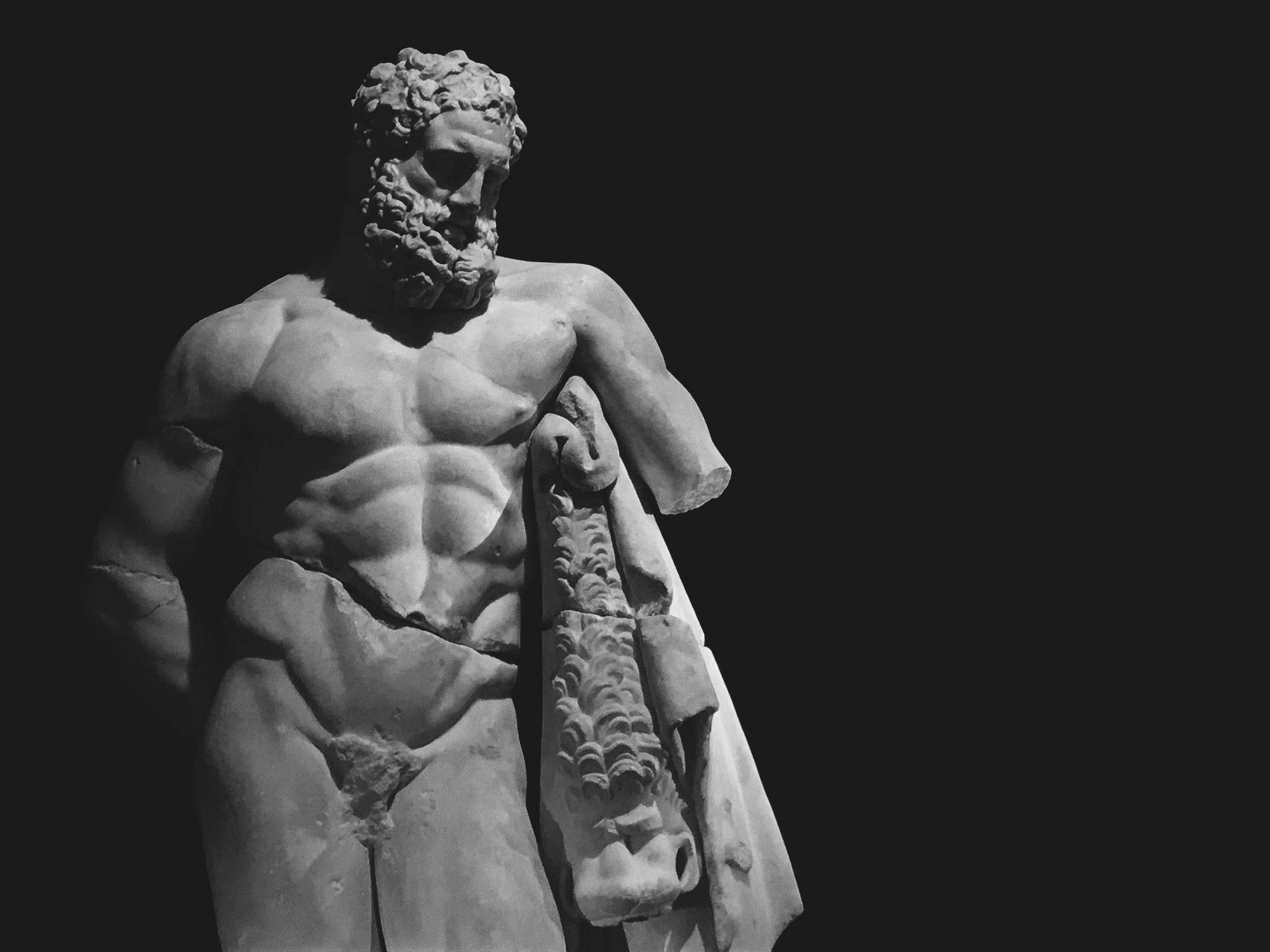
The data meh
My job in this digest is to follow the data news, whether I like it or not. I try to select articles I feel relevant to depict how our field is evolving. Last year, the data mesh trend was strong. This year, facing the reality some big and mature companies applied it, the others forgot it. The mesh, or I prefer, the decentralisation is a great system, but it works only with mature tech and teams. And stars do not align often.
Jean-Georges bet than the next generations of data platforms will be the data mesh. Obviously the articles contains arrows and square because we need processes. But it covers the 4 mesh principles. If you are still sceptical, you can read how Netflix adopted the mesh. Technically their key part is the Kafka cluster allowing the needed decentralisation of a such organisation.
In conclusion I also share this recent article about decentralized data engineering. I feel that the article is hard to read, but it greatly depicts the different phases data eng teams face. From being the central team, then facing shadow it, then generating data silos to become a decentralised team. It embarks so much concepts you need to implement to be successful like self-server, data products, data contracts, etc.
Best practices and learning
This week I've come across a lot of different resources to learn stuff or best practices about data. Here what I've found:
- ❤️ 4 software engineering best practices to improve your data pipelines (I recommend everyone to read it).
- Data documentation best practices — Something fairly simple, or just common sense, but excellent reminder.
- Best practices regarding S3 buckets
- Everyday data science — This is an interactive course about data science (the first lesson is free) ; this is a fun way to learn.
- Amplify Partners hub for data teams — This is a collection of hand-picked linked to create awesome data teams (this is like my links page ; but I know you're waiting for the explorer new version, which is coming soon).
ML Saturday 🤖
The modern data stack is not really the data scientists heaven. This is normal, firstly data teams address the base of the AI hierarchy of needs. But now that we have years of experience in data science with many fails we found way to put in production machine learning. Some people calls it MLOps.
This week Coveo's Director of AI shared how they do MLOps. Jacopo describes their Metaflow usage, from the project startup to the model deployment. I really like the post because it's an overview but greatly depicts how you can integrate ML in the AWS context with dbt and Snowflake.
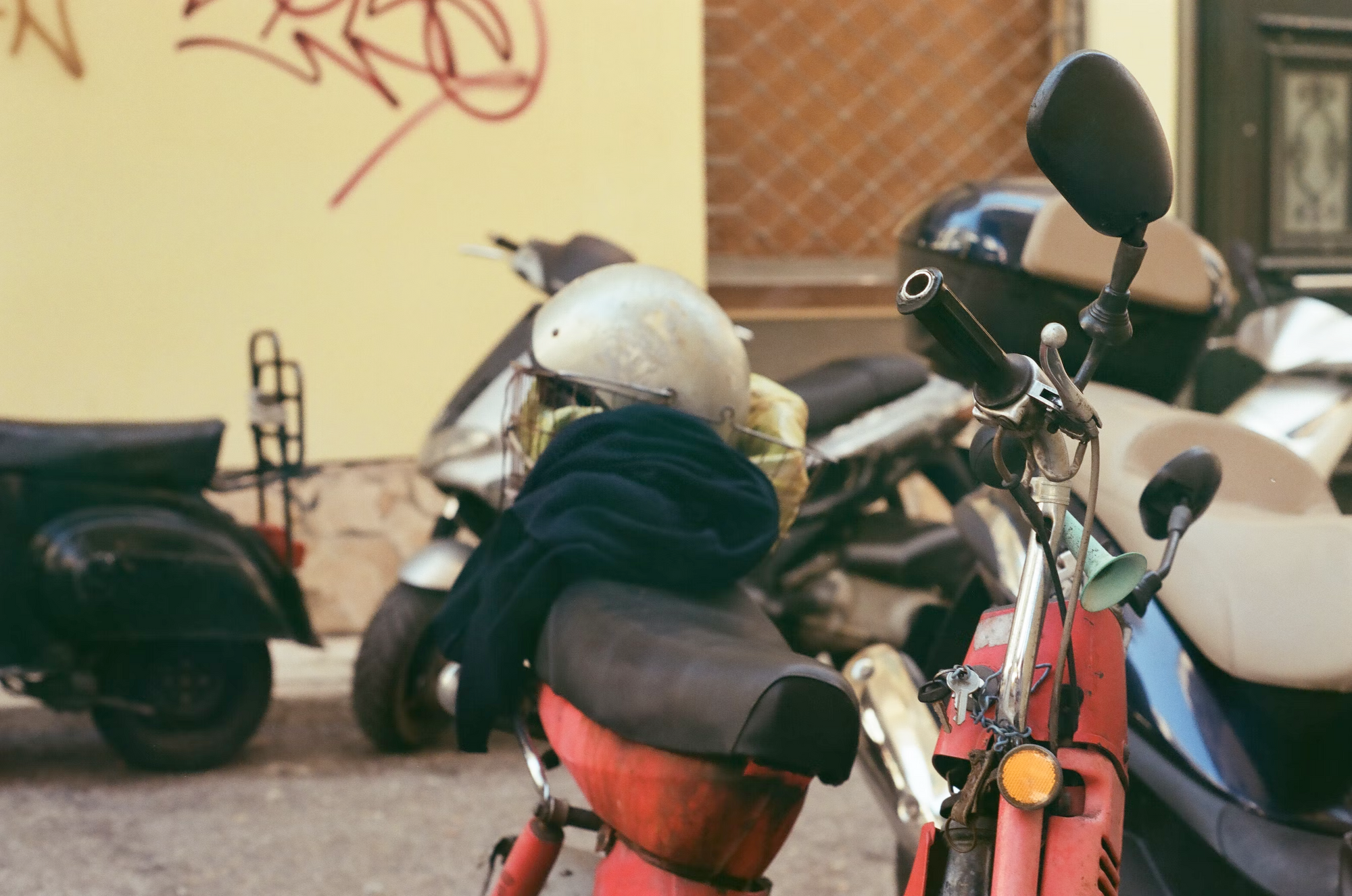
Fast News ⚡️
- dbt Staging highlights — this is like the online demo of the dbt Labs product team. From latest Staging we saw the Python models will be out in v1.3 and that a new Cloud IDE is in the making. Fill the form if you want to apply to the beta program.
- Using Apache ECharts in Python — ECharts has been the Apache JavaScript viz library — sporadically replacing D3 in Superset — and Mark do a walkthrough on how you can use it.
- 📺 Why is Kafka fast? — YT video about Kafka storage specificities that makes it fast. The ByteByteGo channel contains great content.
- How to pick a BI tool — Resilia shared how they pick Preset among Tableau, Looker and Lightdash.
- The cost of product analytics data in your data warehouse — Old post but I like the approach to quantify every work for data resources to justify the buy or build. Even if the post is selling the author tool it's still relevant in the method.
- Dataset-centric visualisations — A podcast with Max Beauchemin and the associated article for people preferring reading than listening. I've been using Apache Superset for the last 3 months and the dataset approach is really refreshing but sometimes annoying.
- 🤓 Building scalable real time event processing with Kafka and Flink — Big technical deep-dive.
- Spark Data Lineage at Yelp & Pricing at Lyft
blef.fr Newsletter
Join the newsletter to receive the latest updates in your inbox.