Data News — Week 23.10
Data News #23.10 — The MAD landscape explained and the Silicon Valley Bank collapse.
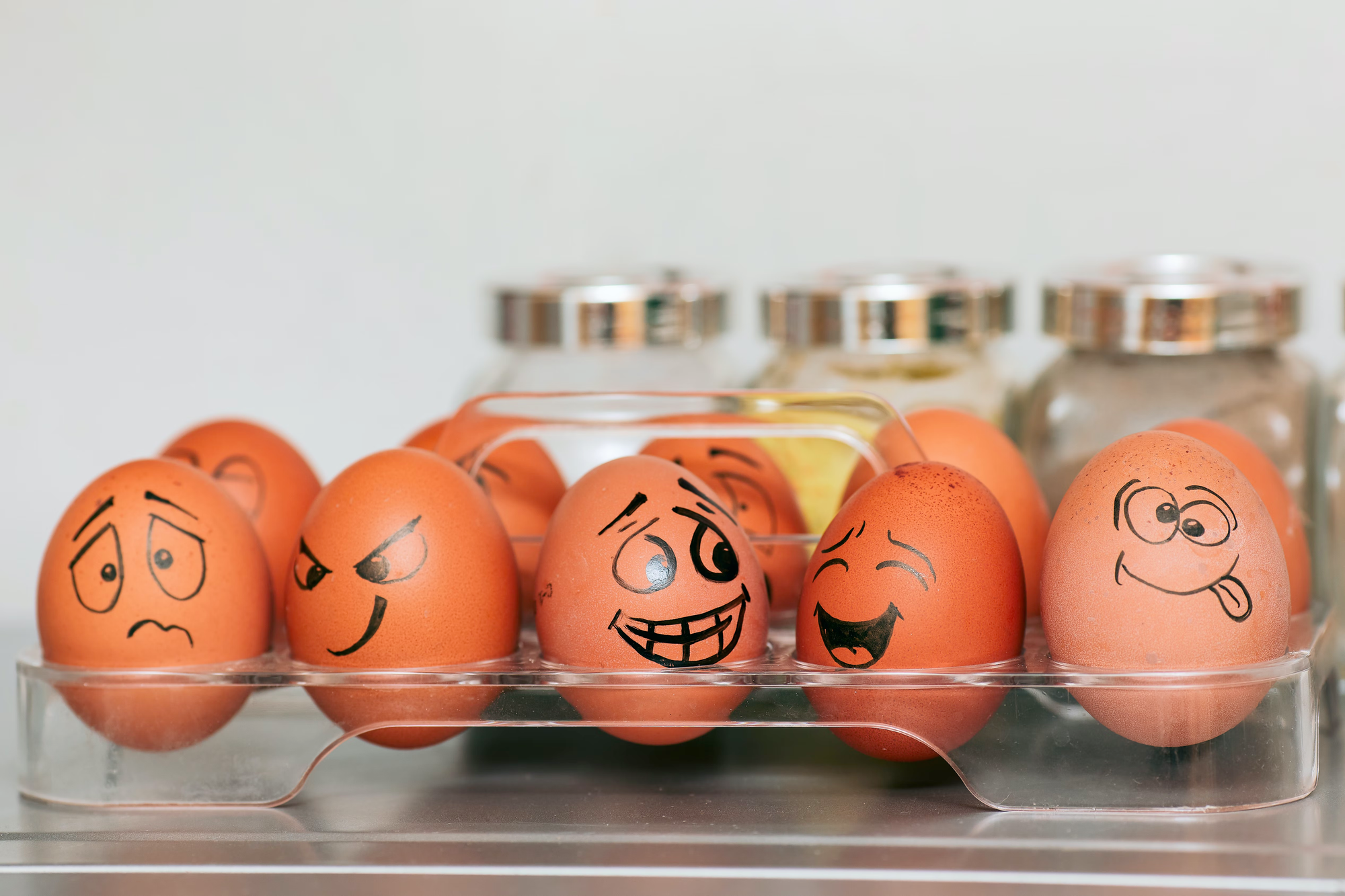
Dear readers, this week Data News lands on Saturday and will be a little bit different than usual because I found less relevant article and as promised last week I wanted to speak about the MAD Landscape.
I hope you will enjoy this topic focus edition where I speak about economics even if I'm a newbie about economy. In last minute I also added stuff about the Silicon Valley Bank that has been seized by the US FDIC, which will generate a crisis in scale-ups/startups world.
The MAD landscape
The Machine learning, Artificial intelligence & Data (MAD) Landscape is a company index that has been initiated in 2012 by Matt Turck a Managing Director at First Mark. First Mark is a NYC VC, in their portfolio they have Dataiku, ClickHouse and Astronomer among other tech or B2C companies.
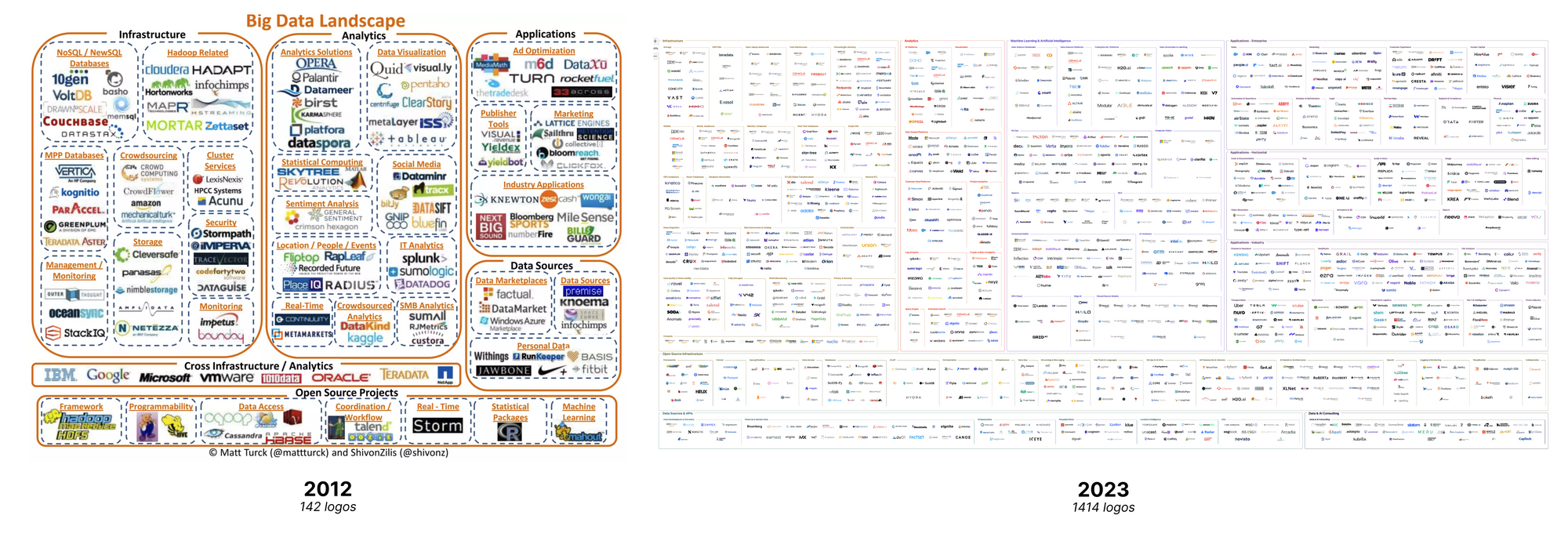
Year after year the MAD Landscape has become an important tool to index the whole data landscape. The choice of categories is also a very clear way to categorise companies and to capture how the data field is changing. Obviously this kind of index is opinionated and they—Matt and his team—make editorial choices when they decide to include—or not—a company, but still, their selection depicts a reality.
Today I want to do a second lecture of the 4-parts article Matt wrote and to give my views on it. As Matt said in a LinkedIn live with Joe Reis the MAD landscape was not published last year (2022) because of time and the landscape has been totally shaken by 2 major events: the massive layoffs wave and the generative AI hype. As a reminder in 2021 edition money was flowing, Databricks did 2 huge rounds with $2.6b raised and Snowflake IPO was a success one year after.
In the MAD landscape we have 3 main parts that I will discuss about today:
- Infrastructure and open source infrastructure — all the data tools everyone wants to use (or not, Talend appears twice in the list 🙃) this part depicts well what a data engineering needs to create a data stack.
- Analytics — this is about the tools we will use to query the data lying down in the infrastructure.
- Machine learning & AI — this category has been totally shaken by the generative AI trend, enterprise machine learning in 2023 is not the same as previously.
Before going more into category changes and macro trends this MAD capture there are a few interesting facts highlighting some biais this index might have:
- 933 companies out of 1414 (65%) are US-based companies
- The continent repartition is 965 (68%) in North America, 182 (12%) in Europe , 74 (5%) in Asia and 192 companies are open-source, so they don't have a base country
- Median founding year is 2015, which means that half of the companies are less than 7 years old, and 20% are less than 3 years old
- GAFAM have logos everywhere. Amazon is the most represented one with 33, then Google with 30 logos, then Microsoft with 21. Apple and Meta are lower with both 2 logos. This is important to mention that IBM have 12 logos and IBM is the oldest company — 1911.
Mainly what these fact are saying is that the MAD landscape is dominated by US-based companies and US-based companies are nowadays thinking how the world should do data, trying to replicate their problem and their vision to everyone. Which is kinda broken. Obviously there are companies or VCs in Europe/Asia but rare are the one with the same impact. Diversity-wise this is a world dominated by the Northern Hemisphere (as always), there is no company in Africa or Southern America for instance.
Key insights
In a nutshell here are the key insights you need to know if you do want to read Matt's notes. First regarding data infrastructure:
- The consolidation will come in the next months/years — every sub-category has between 20 and 30 logos, even if every company think it's unique, they often do the same as other and the market might not be as large as thought. Also there are a lot of "single feature companies" which will compete with broader ones and more likely fails because of offering. Snowflake and Databricks are the adults who will whistle the end of recess.
- Quality and observability are the same — sorry but everyone want to be the "Datadog of data". When looking at the trend they all want to do the same.
- The future of data catalogs is unclear — I really like the definition of catalog Matt gives: "there is a need for an organised inventory of all data assets". Catalogs are still struggling being adopted even if they seem to be asked by a part of the industry. There are also too many alternative.
- With the recession, modern data stack is attacked — This is a big shortcut but true. Modern data stack is tightly coupled to ELT which means load first and think second. When you load first you have more data than you need which leads to avoidable costs. The actual MDS with unlimited computing power and storage might come to an end.
- If you want another perspective with a more exhaustive list of changes you can read Anna's takeaways about MAD 2023 infra category.
After infrastructure Matt also writes about all AI impacts:
- The index this year depicts the generative AI hype with a lot of early stage startup doing almost everything possible with generative algorithms.
- According to Matt we are now in the 3rd cycle AI hype. This is the largest one because it reached mainstream coverage. As a proof my father is using ChatGPT (in French "chat" means "cat" and he says CatGPT, which is a bit funny). But yeah AI became mainstream even if it was already everywhere before, but it was vertical models. But now everyone experiences the general purpose intelligence.
- Startups might have difficulties catching up tech giants on this because they need data and probably a lot of computing power they might not have.
- There are many backlashes AI companies will have to navigate through: impact on job market, algorithm bias, disinformation, hallucination—a word for AI is often wrong, and lastly AI is just boring.
In addition to this O'Reilly released their technology trends from the searches on their website, when we only focus on the data field what we see is:
- Overall Python is the most popular concept and the most growing one — I think it explains because Python is the best entry-level langage for the IT world.
- When it comes to data, data engineering is the most searched concept and growing
- Spark and Hadoop have been less searched than last year
- PowerBI is the 3rd most searched concept and I'm sad about it
Silicon Valley Bank—wat?
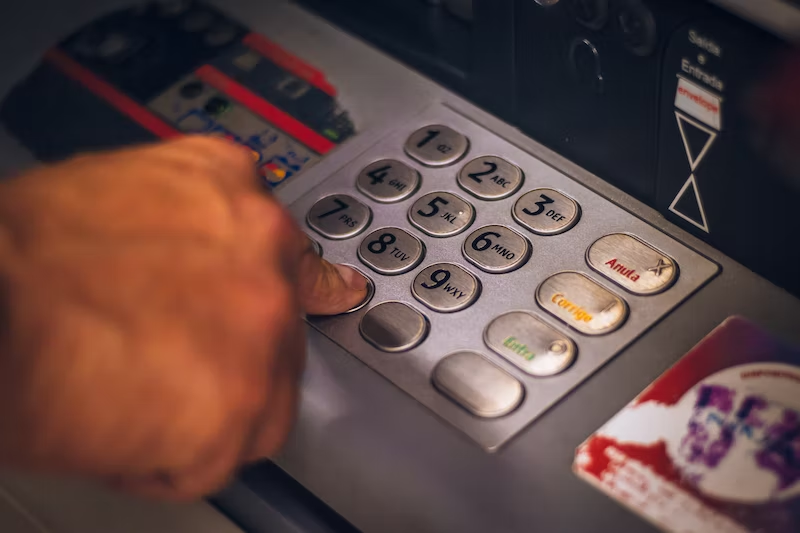
This is a bit last minute but this is freaking huge. Let me do a recap for you and why does it matter.
The Silicon Valley Bank (SVB) is a deposit bank based in California and has the biggest market share. SVB manages billions dollars of assets. Mainly the assets are coming from Silicon Valley startups, founders and employees. In a nutshell if you are a startup founder and you get millions from a seed round you put the money in the SVB.
2-3 years ago a lot of money was raised, the SVB got around $200 billion in deposit. The SVB wanted to put $80 billion of this money at work using Mortgage Backed Securities (MBS)—just as a reminder MBS were at the center of the 2008 financial crisis. MBS guarantees 1.5% return and because interests rates were low because of the pandemic it was ok.
In the last months the FED increased rates crossing recently the 4.5% mark which still was ok. But started triggering chain reaction of all actors. The SVB did a first mistake that I'm not able to explain.
Then VCs started panicking (e.g. Peter Thiel's) advising founders and startups to get the money out of the SVB. Which led to a bank run.
A bank run or run on the bank occurs when many clients withdraw their money from a bank, because they believe the bank may cease to function in the near future
Then the SVB did another mistake. One day later the stock was 60% down and later the same day the bank collapsed.
What happened here is huge and will have a big impact on every US-based scale-ups/startups—it's very well linked to the MAD landscape. Mainly the deposits were only insured until 250k and it means that a lot of companies will lack of cash and probably have difficulties to pay salaries and/or vendors soon.
As a reaction it'll, sadly, imply more layoffs in the coming days and weeks. Other are also afraid of a contagion to the whole banking system as the SVB collapse became the second-largest collapse of the US history.
Data Economy 💰
- Lonestar raises $5m in seed to put data centers on the Moon. Yep, you read it well. Apparently moon market projected to generate $105B in revenue over next decade. While in France we are fighting to retire earlier people wants to send my Twitter history backups to the moon.
- Employees are feeding sensitive business data to ChatGPT, raising security fears.
See you next week with an usual Data News ❤️.
blef.fr Newsletter
Join the newsletter to receive the latest updates in your inbox.